In the high-stakes world of professional tennis, a controversy brewing on clay courts surprisingly affects us all. Just this year, clay courts – the only type of tennis surface that leaves a physical mark where the ball lands – switched to 100% automated, AI-based line calling.
What happens when human eyes all agree the ball is out, but the AI doesn’t? When players can literally photograph the evidence contradicting the machine’s decision?
This seemingly niche tennis controversy offers a perfect microcosm for understanding the challenges we face with artificial intelligence. What happens when AI systems confidently deliver outputs that contradict observable reality? And what does this mean for our AI-integrated future?
When AI Meets Physical Evidence: The Clay Court Dilemma
Until recently, electronic line calling systems were considered virtually infallible. On hard courts and grass, where balls leave no visible mark, players could not verify or challenge the system’s decisions once tournaments moved to fully automated calls.
All that changed this year. With tennis authorities mandating electronic line calling on all surfaces – including clay – we suddenly have a unique situation where AI systems must face direct accountability. Clay, unlike other surfaces, preserves physical evidence of where the ball landed. And this is where the problem emerges.
When Electronic Calls Contradict Physical Evidence
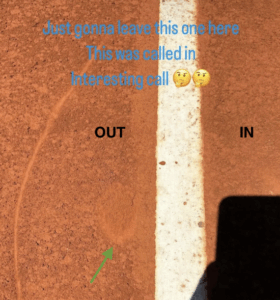
The current controversy stems from a fundamental disconnect: when a ball lands on clay, it leaves a visible mark. If the electronic system says a ball is in but the mark clearly shows it’s out (or vice versa), which should players trust?
This isn’t a hypothetical scenario. At the Madrid Open, world #2 Alexander Zverev was so frustrated by what he believed was an incorrect electronic call that he grabbed his phone, took a picture of the ball mark, and posted it on social media with the caption: “Just gonna leave this one here. This was called in.”
Aryna Sabalenka did something similar at a tournament in Stuttgart. These aren’t isolated incidents – they represent a growing frustration among players who can literally see evidence contradicting what the AI system claims.
What makes this situation particularly interesting is that it creates a perfect real-world test case for AI accuracy. On hard courts, there’s no physical evidence to contradict the electronic call. On clay, the evidence remains visible for anyone to see. It’s the equivalent of an AI image recognition system misidentifying an object that’s clearly visible to human observers.
The Hallucination Problem Goes Mainstream
What we’re witnessing in tennis parallels a challenge AI researchers have grappled with for years: hallucinations. In AI contexts, hallucinations refer to outputs that are confidently presented as factual but have no basis in reality.
We’ve seen high-profile examples of this phenomenon with large language models. Google’s Bard incorrectly claimed the James Webb Space Telescope took the first images of planets outside our solar system. ChatGPT has fabricated legal cases, academic papers, and historical events that never existed.
However, these examples often feel abstract. But tennis provides a vivid, easily understood demonstration of the problem: a ball mark clearly showing one reality, while an AI system confidently asserts another.
What’s particularly concerning is that these systems are designed to provide definitive, unappealable judgments. Once the electronic line-calling system makes a call, players cannot challenge it—the system is presumed to be infallible. This mirrors how many AI systems are being deployed in more consequential domains, where human oversight or appeals processes are limited or nonexistent.
High Stakes Beyond the Tennis Court
If electronic line calling can get it wrong in tennis – where the physics are relatively straightforward and the evidence is visible on the ground – what does this suggest about AI systems making decisions in more complex domains?
Consider applications where the stakes are considerably higher:
– Medical imaging, where AI must detect subtle anomalies in X-rays, CT scans, and MRIs.
– Autonomous vehicles making split-second driving decisions in unpredictable environments.
– Financial systems approving or denying loans based on complex data patterns.
– Legal applications that influence sentencing or bail decisions.
If an AI system can’t reliably determine whether a tennis ball touched a line – a simple yes/no question with visible evidence – how can we trust it to correctly identify a small mass in a lung scan or determine a defendant’s flight risk?
The tennis controversy highlights a crucial question: What happens when AI confidently makes a wrong call, and who has the power to challenge it?
The Canary in the AI Coal Mine
As we rush toward greater AI integration across sectors like healthcare, finance, and criminal justice, the tennis controversy serves as a warning: even the most sophisticated systems can confidently deliver wrong answers. The challenge isn’t just technical but institutional – creating systems that acknowledge their limitations and provide mechanisms for human oversight.
For professional tennis players, an incorrect line call might cost them a match point. For someone receiving an AI-assisted medical diagnosis or legal decision, the stakes are immeasurably higher.
When players like Zverev pull out their phones to document AI errors on clay courts, they’re not just protecting their competitive interests – they’re demonstrating a problem we all need to take seriously before we surrender more critical decisions to systems that can hallucinate with confidence.